文章目录(Table of Contents)
简介
在上一篇中,Transformer 结构介绍,我们详细介绍了 Transformer
中的每一个模块。这一篇会完整过一遍整个 Transformer
,也是因为看到一个非常好的关于 Transformer
图解的视频,所以想在这里进行记录。这里的可视化内容会将自注意力和位置编码,多头注意力,注意力分数,全部再一次进行解释,进一步加深理解。
本文的所有内容全部参考自视频,Illustrated Guide to Transformers Neural Network: A step by step explanation。我这里只能把视频中关键的内容进行摘录,非常推荐大家去观看原始的视频。
Transformer 结构
Transformer
是一个 Encoder-Decoder
的结构。这里我们通过一个「对话系统」来对 Transformer
的结构进行详细的图解。我们希望输入是「Hey, how are you ?」,输出是「I am fine.」
如下图所是,Encoder
会首先从句子中提取信息,输入是「Hey, how are you ?」,输出为该句子对应的信息:
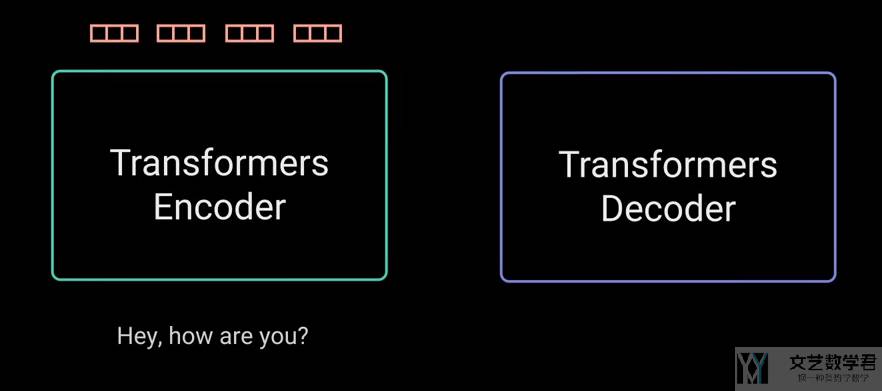
接着 Decoder
会根据 Encoder
的输出,和 Decoder
的输入,依次返回每一个单词。这里返回的是「I am fine.」。
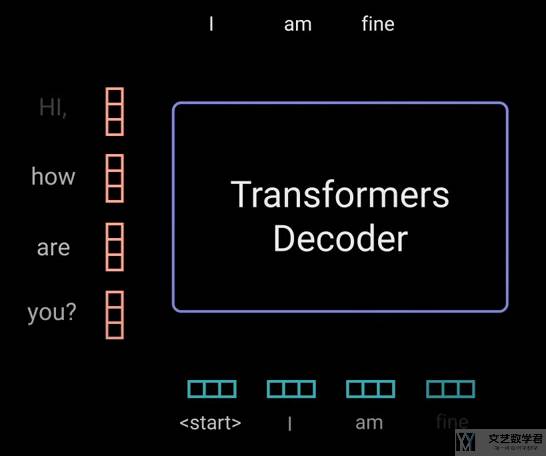
下图是 Transformer
一个完整的工作流程,包括 Encoder-Decoder
中详细的模块。在 Decoder
中是有一个循环,会将每次的输出作为下一次的输入。
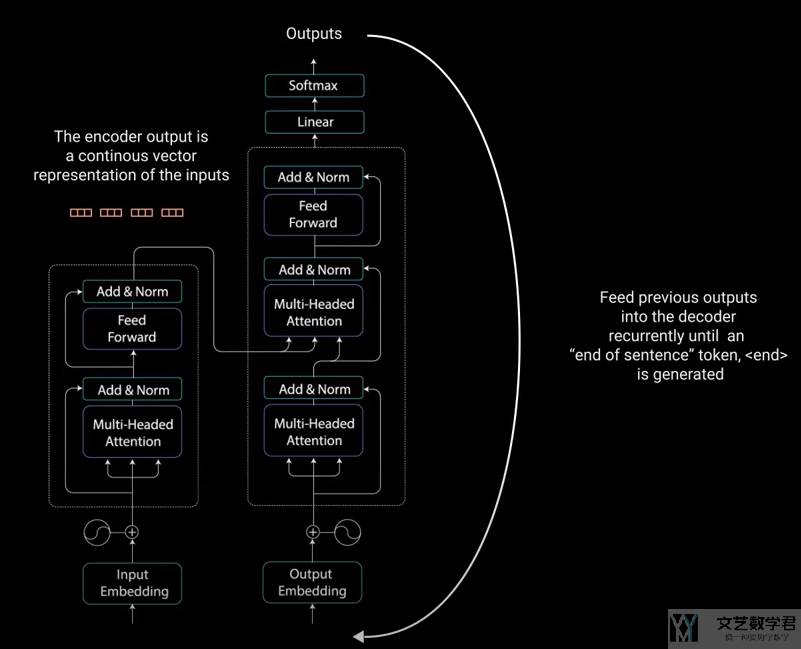
至此我们对 Transformer
有了一个非常粗略的了解。下面来详细看一下内部是如何运作的。我们还是会分别介绍 Encoder
和 Decoder
这两个部分。
Encoder 部分
Encoder
的目的是「encode the input into a continuous representation with attention information」。这个可以帮助 Decoder
聚焦在重要的词汇上。
Input Embedding
这一部分会将文字转换为一个连续的向量,如下图所是:
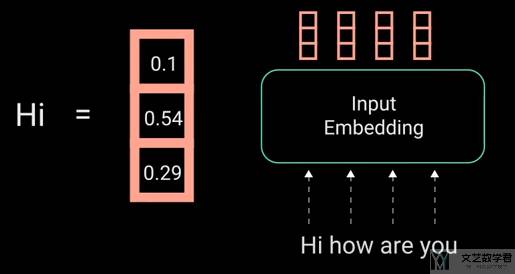
Positional Encoding
接着需要对「input embedding」的结果加入「位置编码」。因为 Transformer
不像是其他 RNN
网络,对输入的顺序敏感,因此需要加入关于位置的信息。如下图所是,我们通过 sin
和 cos
函数来加入位置信息:
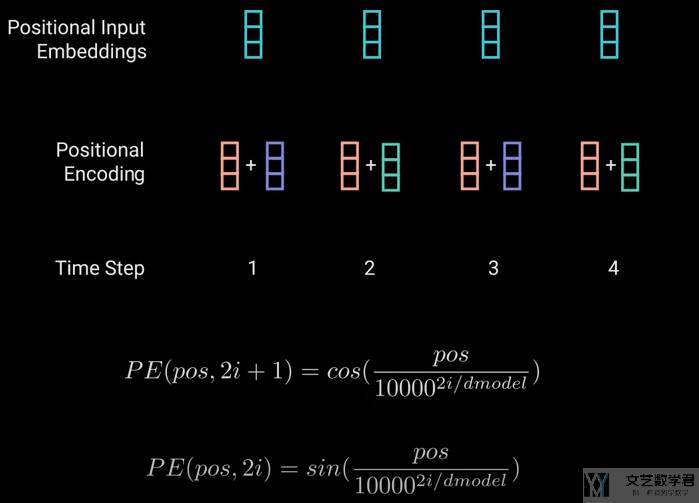
Encoder Layer
接下来是 Encoder Block
的部分。主要会有两个部分组成,分别是「Multi-header Attention」和「Pointwise Feed Forward」。这两个部分使用「residual connection」进行连接。这部分的主体结构如下所是:
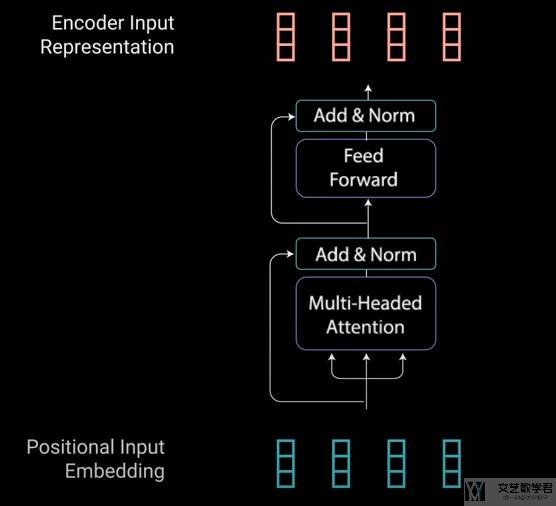
且 Encoder Block
是可以重复 N
次,来进一步加强模型的表达能力:
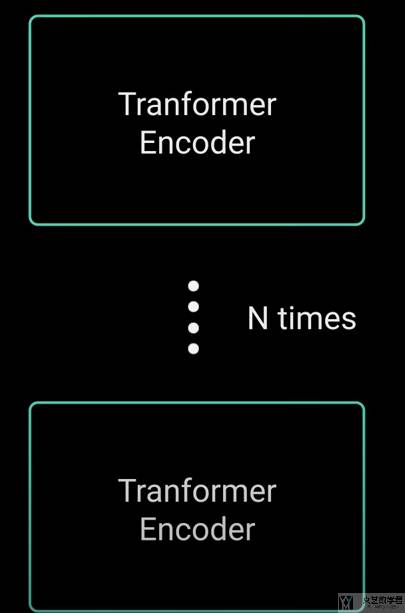
Self-Attention
在介绍 Multi-header Attention
之前,首先介绍 self-attention
的机制,该机制使得输入中的每个单词与输入中的其他单词相关联(associate each individual word in the input to other words in the inputs)。self-attention
的计算流程如下所是:
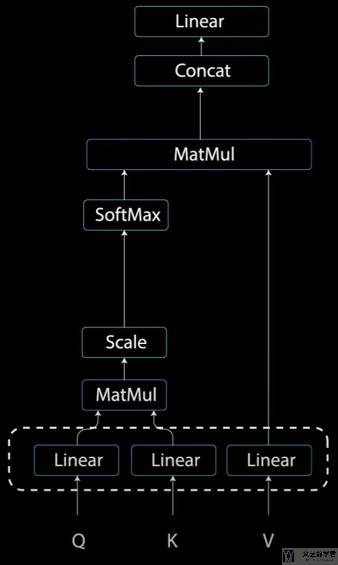
我们首先将「input」输入三个不同的全连接层,来生成 key
,value
和 query
。
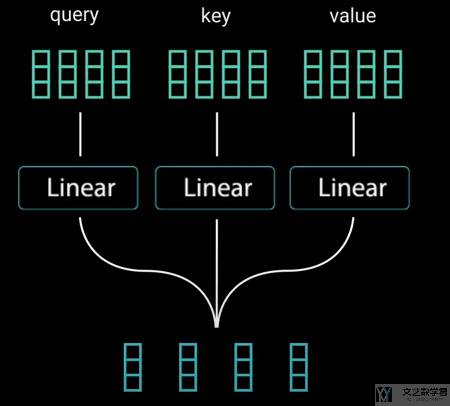
接着根据计算得到的 key
和 query
,计算单词两两之间的得分(对应self-attention
的计算流程图中的 Matmul
部分)。
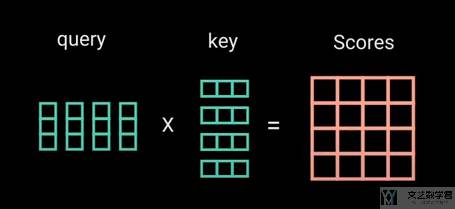
这里 scores matrix
表示一个单词对另外的单词有多大的重要度,关联度。
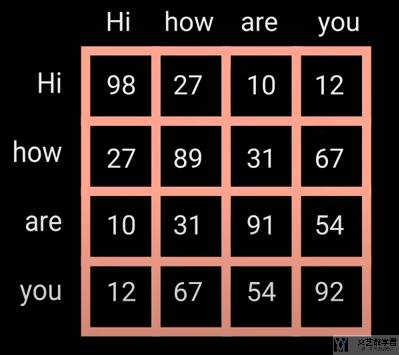
因为上面的值的大小与句子长度有关,因此我们需要对其进行标准化,除 query
的长度(对应self-attention
的计算流程图中的 Scale
部分):
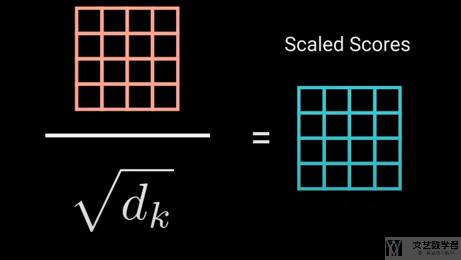
接着通过 softmax
来计算 attention weights
,通过计算 softmax
,可以使得大的数字变得更大,小的数字变得更小(对应self-attention
的计算流程图中的 Softmax
部分):
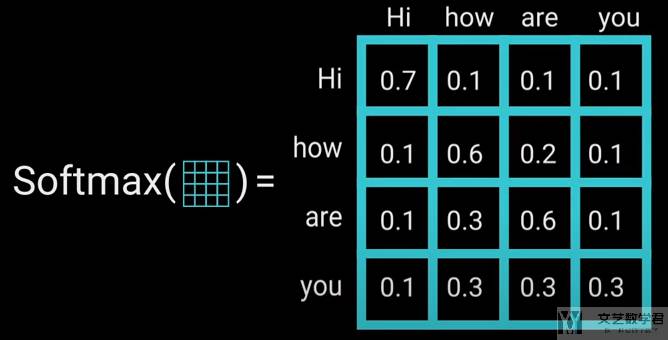
接着将上面计算得到的 attention weights
与 values
相乘,此时相当于是对 values
进行了一个加权相加的操作(对应self-attention
的计算流程图中的 MatMul
部分):
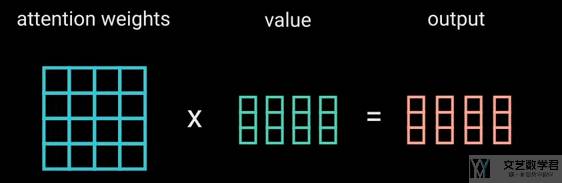
最后将上面 outputs
的输出通过一个全连接层即可(对应self-attention
的计算流程图中的 Linear
部分):
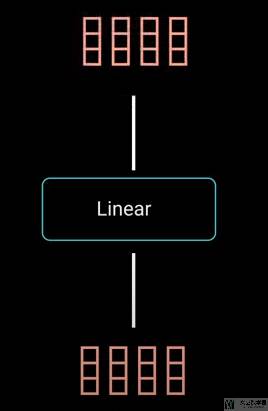
Multi-header Attention
有了上面的 self-attention
的结构,我们希望模型变得更加 power,此时可以在在开始的步骤,使用不同的全连接网络来计算key
,value
和 query
。接着每一个部分按照上面 self-attention
的步骤进行计算。
这里每一个 self-attention
称为是一个 head
。对每一个 head
的输出,我们对其进行合并,形成一个新的特征:
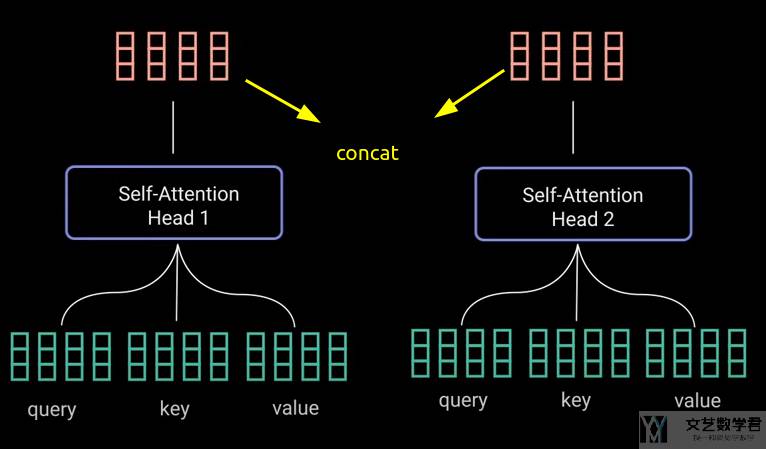
Pointwise Feed Forward
对于 multi-head attention
的结果,在通过 Layer Norm
之后会进入 Pointwise Feed Forward
,这个其实就是两层全连接网络,对特征维度进行变化(give it a richer representation)。
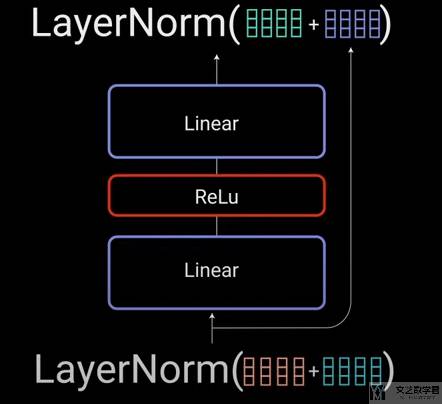
Decoder 部分
这部分开始介绍 Decoder
的部分。Decoder
的目的是产生文字序列。Decoder
的结构其实与 Encoder
是类似的,包含两个「multi-head attention
」和一个「Pointwise Feed Forward
」。
Decoder
部分是一个 auto regressive
,他会将之前的输出作为输入。在预测 <END>
之后,则会停止进行预测。下面对 Decoder
部分进行详细介绍。
同样,Decoder
部分也是可以由多个 Decoder Layer
堆叠组成,且可以与 Encoder
部分进行不同的组合,即第二个 multi-head attention
中的 key-value
是来自 Encoder
的第几层。
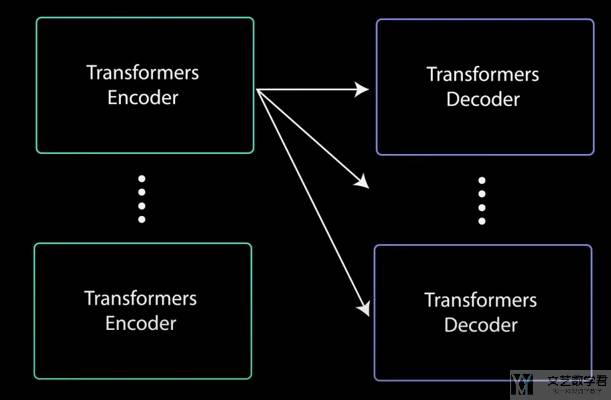
Output Embedding & Positional Encoding
与 Encoder
部分类似,我们将对话的文字进行 embedding
和加入位置信息。这里训练的时候我们相当于是知道回答的句子的。
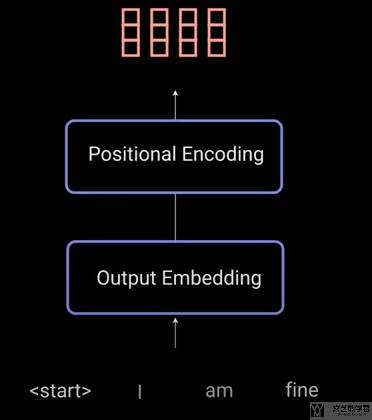
Decoder Multi-head Attention-1
接下来我们看 Decoder
部分的第一个 multi-head attention
。与前面 Encoder
部分的有一点区别,就是这里需要加上 mask
。例如现在模型才输出到「am
」,那么 attention
的信息中是不能包含 「fine
」 的信息的,不能让模型提前看到答案。
于是我们可以像下面这样加上一个「Look-Ahead Mask
」来遮住后面单词的 attention score
,这一步骤在计算 softmax
前面进行。
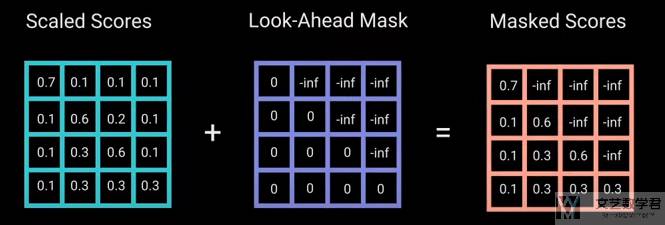
这样「-inf
」在计算 softmax
之后就会得到结果 0
。
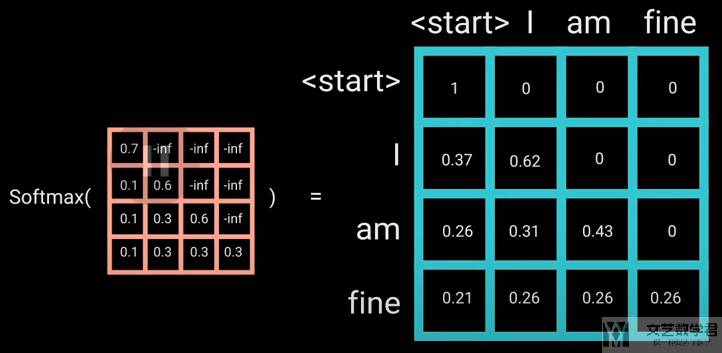
Decoder Multi-head Attention-2
对于 Decoder
部分的第二个 multi-head attention
,这里就不再是 self-attention
。他的 key-value
是来自 Encoder
的输出,query
是来自前面一个 multi-head attention
的输出。这里是使得模型考虑 Encoder
的输出与当前哪一部分是有关的(decide which encoder input is relevant to)。
接着第二个 multi-head attention
的输出会通过 Pointwise Feed Forward
进行进一步的处理,这个与前面 Encoder
的部分是一样的。
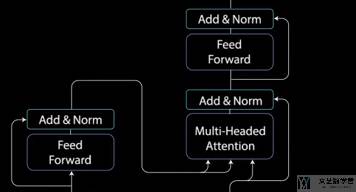
Linear Classifier
Decoder
的最后一个部分是 Linear+Sotfmax
,这里会输出每个单词的概率。例如现在有 10,000
个单词,最后一层的输出就是 10,000
。
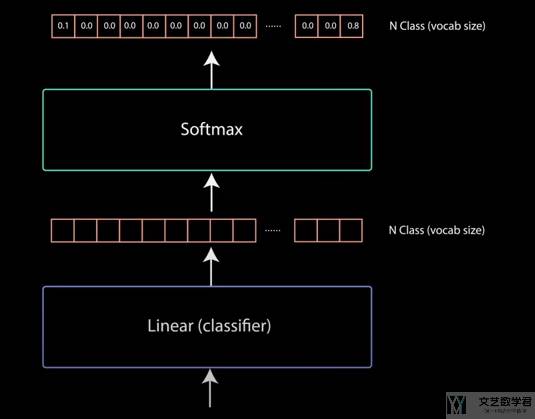
- 微信公众号
- 关注微信公众号
-
- QQ群
- 我们的QQ群号
-
评论