文章目录(Table of Contents)
简介
这一篇会介绍一些强化学习在工业界的应用. 学习一下如何将强化学习的思想用在日常的生活中去. 这里主要会介绍使用强化学习去控制交通灯.
IntelliLight: a Reinforcement Learning Approach for Intelligent Traffic Light Control
这一篇是使用强化学习来调节信号灯. 文章的具体信息如下:
- Wei, Hua, Guanjie Zheng, Huaxiu Yao, and Zhenhui Li. "Intellilight: A reinforcement learning approach for intelligent traffic light control." In Proceedings of the 24th ACM SIGKDD International Conference on Knowledge Discovery & Data Mining, pp. 2496-2505. 2018.
这篇文章有一个介绍视频, IntelliLight: a Reinforcement Learning Approach for Intelligent Traffic Light Control
关于使用强化学习来控制信号灯, 有下面几个点可以重点考虑一下.
- 一个周期内红绿灯的总时长是一样的, 应该如何控制;
- 一个路口的交通灯如何不冲突. 两个道不能同时绿灯;
- 如何考虑多个路口, 多个路口的红绿灯实现联动.
目前存在的问题
While existing traffic lights are mostly operated by hand-crafted rules, an intelligent traffic light control system should be dynamically adjusted to real-time traffic. (目前大部分的信号灯系统还是手动进行调整的)
However, existing studies have not yet tested the methods on the real-world traffic data (目前的方法没有使用实际的数据进行测试)
They only focus on studying the rewards without interpreting the policies. (同时, 目前的研究值去比较reward, 但是没有对生成出来的策略进行比较. 这个会在创新点的地方详细的说明)
本文创新点
本文的创新点主要是下面三个点:
- Experiments with real traffic data. (实验中使用真实的数据)
- Interpretations of the policy. (通常, 我们衡量一个信号灯控制系统的好坏会使用overall reward, 但是之前很少会有研究去看得到的策略. 例如下图中, 这两个策略的overall reward是相同的, 但是在实际使用的时候, policy 1会更加好, 因为更加稳定)
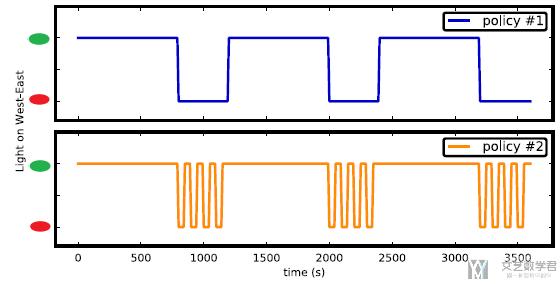
- A phase-gated model learning. (之前我们说到, 当前信号灯的状态会作为一个特征来输入模型. 但是实际上单一的特征通常对模型的结果没有重要的影响. 例如下图, 道路状态是一样的, 只有交通信号灯不一样, 但是模型很可能给这两个场景同样的action, 改变当前或保持不变. 这篇文章提出new phase-sensitive来解决上面的问题) 我们也可以理解为, 除了state之外, 我们还会考虑不同的phase, 对于不同的phase训练不同的模型. 这个会在下面网络结构部分详细说明.
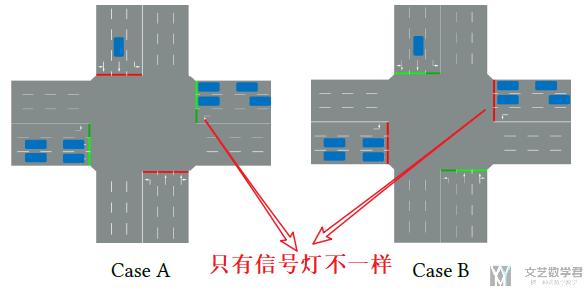
使用强化学习来解决信号灯管理概览
使用deep reinforcement learning来解决信号灯的管理, 主要结构如下图所示:
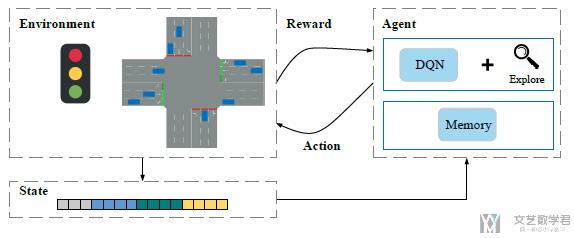
其中:
Environment由两个部分组成, 分别是traffic light phase(信号灯的状态)和traffic condition(目前交通的状况). State就由上面两个组成的特征构成.
Agent(控制信号灯系统的, 可以理解为就是交通灯)将state作为输入, 来学习在当前的state下, 是"keep the current phase of traffic lights(保持当前的信号灯)", 还是"change the current phase (修改当前信号灯)"
接着这个decision会发给环境, 环境此时会返回一个reward和新的state. 这样就是一整个过程.
详细说明强化学习环境
下面我们对强化学习中各个状态做一下定义. 这里我们考虑的是一个路口(intersection)的一个信号灯.
- state
- traffic light phase
- Green-WE (此时West到East方向是绿灯, 那么South到North方向就是红灯, 只需要确定一个方向的信号灯即可)
- Red-WE
- traffic condition (这里每一个都是针对每条路的, 比如一个路口有四条路相连, 那么一个特征就会有4个值. 比如道路1上汽车等待长度, 道路2上汽车等待长度.)
- queue length (车道的汽车等待长度, L), 比如路口有4个车道, 这里就会有4个值.
- number of vehicles on the lane (车道上的汽车数量, V)
- updated waiting time of all vehicles on the lane (一辆车的等待时间, W). 例如一辆车在0-15s的时候速度为0.1m/s, 在15-30s的时候为5m/s, 在30-60s的时候为0.1m/s. 这个时候W在这三个时间点(t=15, t=30, t=60)分别是15, 0, 30.
- 摄像头的图片信息也会传入.
- traffic light phase
- action (只有两个action, 分别是保持当前状态和改变当前状态)
- a=1, change the light to the next phase;
- a=0, keep the current phase
- reward (reward计算主要由下面几个因素影响)
- 将各个道路的等待车辆长度(L)求和(当速度小于0.1m/s的时候, 认为是在waiting)
- 所有车道的waiting time(W)的求和. 相当于对所有等待的车的waiting time进行求和.
- C表示信号灯颜色是否改变, where C=0 for keeping the light, and 1 for changing the light.
- delay of lane (D). D的计算公式为: 1-LaneSpeed/SpeedLimit; 其中LaneSpeed表示这个车道目前的车速, SpeedLimit表示这个车道的最大速度. 也就是越堵车, 这个D会越接近1.
- 在时间t内, 通过路口的车辆数量N.
- 所有车辆通过路口时的total travel time, T.
最终reward的计算如下所示(注意都是求和的, 把所有的lane的数据进行求和):
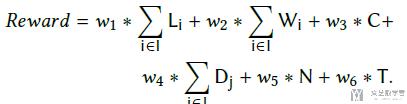
其中所有的系数如下所示:

我们可以简单分析一下: 等待车辆长度越长, 等到时间越长, 信号灯的经常改变, delay of lane越大, 此时reward是越小的. 但是在给定时间内通过路口的车辆数量越多, 行驶的时间越长, reward是越大的.
深度强化学习网络结构
下面, 介绍整个模型的结构. 如下图所示, 分为两个部分, offline part和online part.
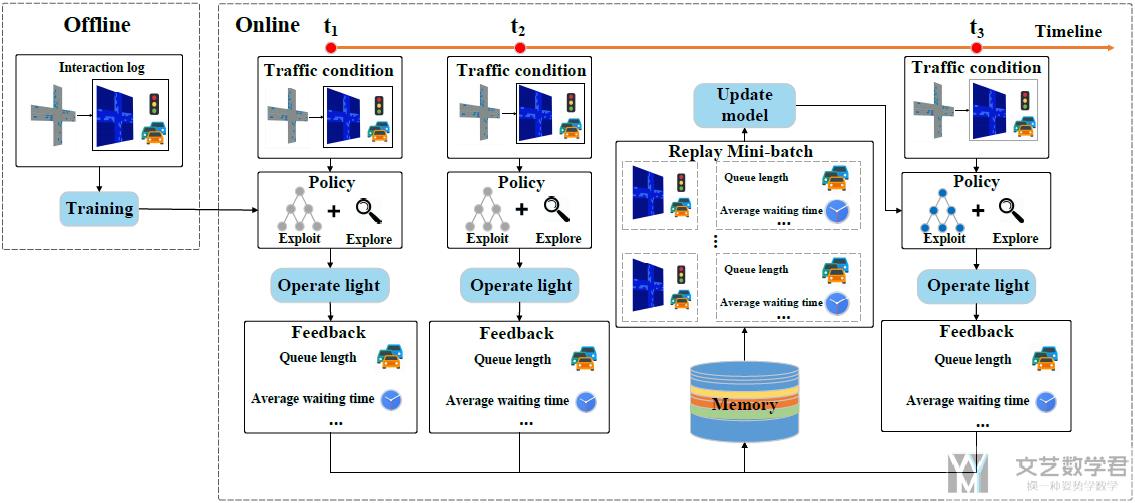
- 在offline part, 我们会使用log来训练系统.
- 在online part, 每个时间t系统会得到一些state(例如我们想每5s确定一下是否要改变信号灯的状态), 接着模型根据这些state给出action, 并得到reward. 我们将这些存入memory. 在一些步骤后, 更新模型.
接着我们详细说明一下network的结构(deep Q-network的结构). 注意下面的结构是包含"Phase Gate"的. 也就是对不同的phase, 会有一个选择, 使用不同的网络. 突出特征phase的重要性. 例如当当前的state完全一模一样, 模型很可能给出的action也是一样的. 但是实际情况下, phase对模型的判断是很重要的, 要单独拿出来.
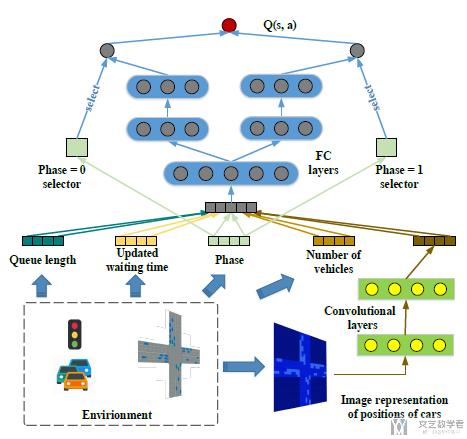
简单对上面的网络结构进行说明.
- 模型的输入由两个部分组成, 一个是道路的状态, 是由数字特征组成. 另一个是通过CNN提取图像特征. 接着将这两部分的特征进行合并(the concatenated features), 输入全连接网络.
- 在模型训练的时候, 会有一个"gate", 针对不同的phase训练不同的网络. 如上图所示, 当Phase=0的时候, 左侧的模型被激活; 当Phase=1的时候, 右侧的模型被激活. (这样做的目的是在不同的phase下, 做的决定可以有区分)
这个模型在解决数据不平衡的问题上, 也是提出了一种方法, 叫做"memory palace". 因为在deep q-network训练的时候, 我们会有一个"experience replay"(memory), 我们会从里面进行抽样来进行训练. 但是如果数据是不平衡的(不同道路的车辆可能是不平衡的), 抽样结果也会是不平衡的.
于是, 本文会使用多个memory, 将不同的phase-action的组合的样本存在不同的memory里面. 具体的结构如下图所示, 在抽样的时候, 我们会从不同的memory里面抽取同样多数量的样本:
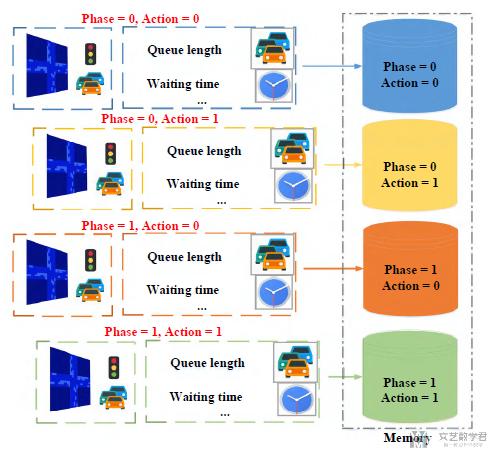
实验结果说明
在本文中, 作者使用了两类数据集进行实验, 分别是使用SUMO生成的模拟数据和真实数据. 同时作者还与其他三种方法进行比较. 同时作者也对自己的方法, 有三种不同的变体进行比较. (是否包含"phase gate", 和是否包含"memory palaces")
首先是在虚拟数据上的结果展示(reward的结果说明), 对于6种方法结果的比较, 可以看出文章提出的方法会比较好.
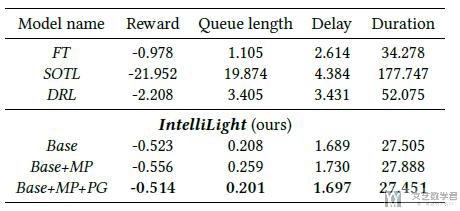
当然, 这篇文章除了对reward进行说明, 还会对生成的policy进行说明. 例如在WE方向上车辆数量变多, 那么我们希望可以动态的调整信号灯的时间, 使其在WE方向上的绿灯时间变长. 于是下图中, 我们的策略可以和事实的交通状况吻合.
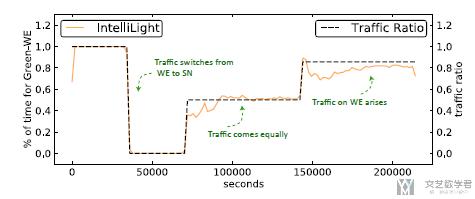
接着是在真实数据上进行测试, 关于数据上的比较我就不截图了, 就是提出的方法会有明显的提示. 我们重点看一下作者在policy上的解释. 首先在一个路口的SN和WE方向上的车流变化如下图所示:
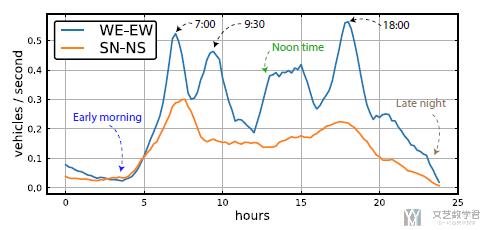
我们给出的策略如下所示, 纵轴表示WE方向上绿灯的时长. 可以看到大部分时间WE方向上绿灯时间长, 在early moring的时候, 由于SN方向的车多, 所以在实际控制的时候也是SN方向绿灯时间长. 可以看到这个模型是可以根据车流进行实时调节的.
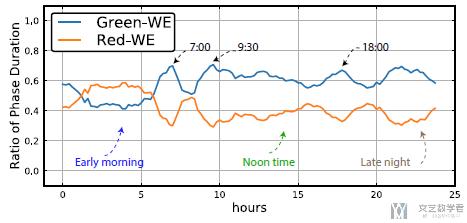
同时, 作者还绘制了车流来的数量与红绿灯变化的关系. 蓝色的虚线表示SN方向的车流. 红色的虚线表示WE方向的车流. 这里其实是讨论该模型会更偏向于主路. 在这里WE方向是主路, 可以看到中间SN方向有车来, 但是模型并没有马上切换信号灯.
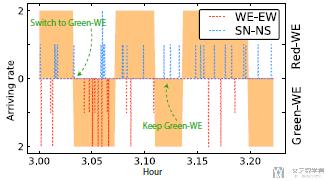
Reinforcement learning-based multi-agent system for network traffic signal control
这一篇是使用强化学习(reinforcement learning)来控制交通信号灯. 这一篇主要介绍交通信号灯之间的协作. 文章的具体信息如下所示:
- Arel, Itamar, Cong Liu, Tom Urbanik, and Airton G. Kohls. "Reinforcement learning-based multi-agent system for network traffic signal control." IET Intelligent Transport Systems 4, no. 2 (2010): 128-135.
一句话概括如何在不同交通灯之间协作: 让不同路口的信息可以在不同的交通灯之间进行共享.
本文的创新点
- We utilise a multi-agent setting, whereby RL is employed as means of controlling the different intersections in the network. (使用一个multi-agent的强化学习模型, 来同时控制多个路口)
- The coordination factor among the intersection is an obscure object and has a nonlinear relation. The AI intelligent algorithm can compute the inner nonlinear relation which cannot be provided by the traditional approach. (多个路口之间的相互影响是复杂的, 我们使用RL来解决)
- Simulation results clearly indicate that the proposed RL control scheme outperforms the LQF (longest-queue-first) algorithm strategy by yielding a lower delay and cross-blocking frequency, particularly for medium and high traffic arrival rates. (实验结果表明使用RL的效果比传统方法LQF要好)
多个路口同时控制的目标
在说明目标之前, 我们先定义一些术语:
- Traffic throughput (交通吞吐量): average number of vehicles per unit of time successfully traverse the intersection.
- Traffic congestion (交通拥堵): typically occurring in multi-intersection settings, is a condition in which a link is increasingly occupied by queued vehicles. Highly congested intersections often cause cross-blocking whereby vehicles moving upstream fail to cross an intersection due to lack of queuing positions at a designated link.
所以, low traffic throughput 和 high traffic congestion会造成交通拥堵. 所以本模型的目标就是最大化traffic throughput.
多个路口环境说明
接着看一下本文所使用的环境. 环境如下图所示:
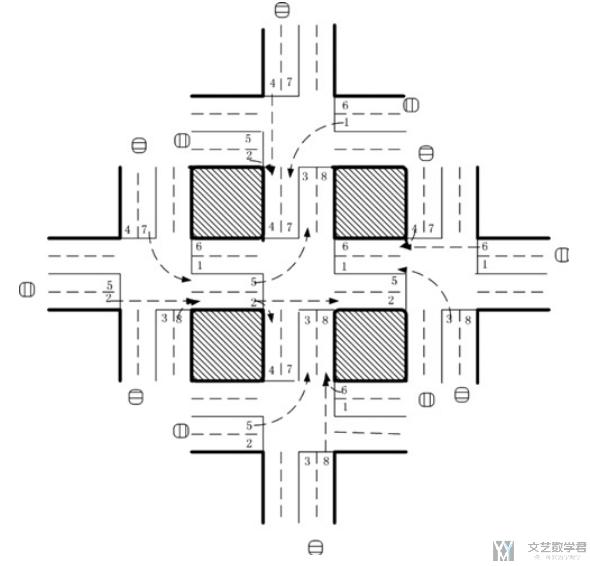
我们简单对上面的环境进行说明:
- 环境描述
- 环境中有5个十字路口, 中间的十字路口是中心路口(central intersection).
- 周围的4个路口被叫做outbound intersections.
- 图中车道数字的含义
- 图中的even number (偶数车道), 车可以直行和右转.
- 图中的odd number (奇数车道), 车可以左转到指定的车道.
- 环境的其他信息
- 一个车道最多有40辆车在进行排队.
- 一个车在离开前, 最多经过一个路口, 或是三个路口(outbound-central-outbound)
- 在multi-agent系统中, 一个agent只能获得他直接的临近位置的信息.
- 在模拟的过程中, new vehicles服从Poisson process来产生. 产生的位置在outbound intersection. 每次排在队伍的最后面.
强化学习每一个元素的对应
- System state
- 从上面的环境介绍的图中可以看到, 一个路口有8 lanes. 于是, 对一个十字路口的state是一个8维的向量, 每一个维度是每一条路的relative traffic flow. (the relative traffic flow is defined as the total delay of vehicles in a lane divided by the average delay at all lanes in the intersection.)
- 对于四个周围的十字路口(outbound intersection agent), 只考虑local traffic statistics.
- 对于与中间的十字路口(central intersection), 他可以获得周围四个outbound intersections的信息.
- Action set
- 在上面的图中, 对于每个lane进行编号, 从1-8.
- 一共有8种action, 分别是{(1,5), (1,6), (2,5), (2,6), (3,7), (3,8), (4,7), (4,8)}. 例如(1,5)表示lane 1和lane 5可以同时通行, 不会有冲突.
- Reward function
- 首先, 我们说一下delay的计算方式: In a real-life application, vehicle delay could be estimated through the application of technological advancements in the field of vehicular sensors and traffic controllers. Advance detector (upstream detectors) actuations can be used to track vehicular arrivals at each intersection approach over time (路口到达的车). Phase change data and saturation headway data can be used to estimate the number of departures from the stop bar over time (路口离开的车). The two flow profiles can then be combined to estimate the queue accumulation on the intersection approach. (到达-离开=队伍长度) The time in queue can be used to estimate delay for each approach and consequently for the entire intersection (queue中每一辆车的等待时间, 就是路口的等待时间).
- 如果当前的delay比过去一段时间的delay要低, 那么reward是正的.
- 如果delay比过去一段时间有所增加, 那么reward是负的.
- 于是reward的定义如下图所示, 其中D_last是过去这个路口的delay; D_current是当前这个路口的delay. 对于central intersection的reward, 需要把周围路口的reward也算进来, 加权.
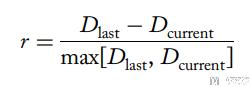
模型的具体设计
在本实验中, 作者只在central intersection中用到了deep Q network. 因为central intersection需要考虑周围的信息, 所以网络的input是5*8=40, 整个网络的结构是40->25->8. 最后output是8维, 是因为一共有8种actions.
实验结果表示
我们比较了LQF方法和MAS Q Learning的方法. 横轴表示到达率, 可以看到随着汽车越来越多(到达率上升), 此时delay也在上升. 但是当车流量大的时候, MAS Q learning的delay就会比较小.
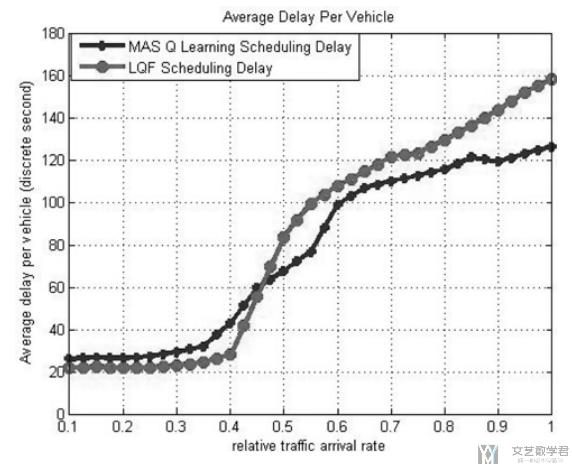
DeepMind AI Reduces Google Data Centre Cooling Bill by 40%
这一篇好像不是论文, 只是google放在deepmind网站上的一篇说明. 原文的链接为, DeepMind AI Reduces Google Data Centre Cooling Bill by 40%. 在这里就简单做一下说明.
Google's data centres一直致力于减少能源的使用, 并使用再生能源. (目前可以做到的是, compared to five years ago, we now get around 3.5 times the computing power out of the same amount of energy, and we continue to make many improvements each year.)
在google's data centres中, 一个很大的能源消耗来自于制冷(cooling). 我们需要使用多种设备, 例如pumps, chillers and cooling towers来降低数据中心的温度. 但是很难很好的控制好这些设备:
- The equipment, how we operate that equipment, and the environment interact with each other in complex, nonlinear ways. Traditional formula-based engineering and human intuition often do not capture these interactions. (设备之间会有相互影响, 但是这些影响不容易被表达出来)
- The system cannot adapt quickly to internal or external changes (like the weather). This is because we cannot come up with rules and heuristics for every operating scenario. (系统不能对外界的变化给出快速的反应)
- Each data centre has a unique architecture and environment. A custom-tuned model for one system may not be applicable to another. Therefore, a general intelligence framework is needed to understand the data centre's interactions. (每一个数据中心都有不同的结构与环境, 如果对每一个单独设置太麻烦了, 所以希望可以有一个统一的框架来实现)
于是, 为了解决上面的问题, 我们使用各种传感器数据来训练一个网络 (taking the historical data that had already been collected by thousands of sensors within the data centre - data such as temperatures, power, pump speeds, setpoints, etc. - and using it to train an ensemble of deep neural networks.) 目标是改善能源利用效率, 使用average future PUE (Power Usage Effectiveness), which is defined as the ratio of the total building energy usage to the IT energy usage.
除此之外, 我们还会训练两个网络, 来预测数据中心的温度和压力变化, 来对之后的action做出模拟, 来确保不会做出一些有问题的操作.
最后将模型放在线上进行使用, 可以看到上面PUE的变化. 使用该系统可以大大增加能源利用效率.
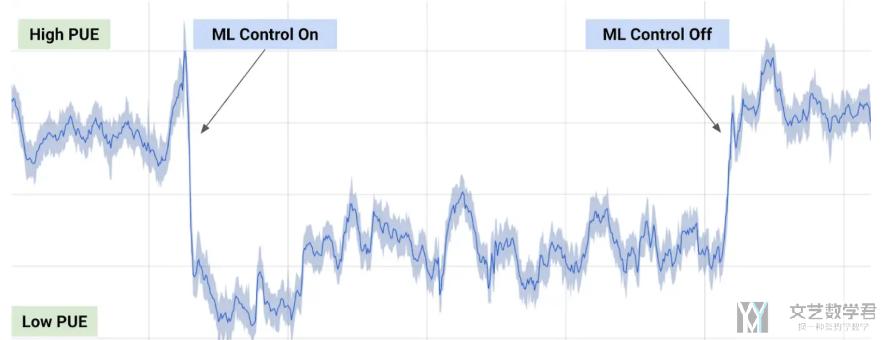
Our machine learning system was able to consistently achieve a 40 percent reduction in the amount of energy used for cooling, which equates to a 15 percent reduction in overall PUE overhead after accounting for electrical losses and other non-cooling inefficiencies. It also produced the lowest PUE the site had ever seen. 同时, 该算法还可以被用在不同的系统上面.
- 微信公众号
- 关注微信公众号
-
- QQ群
- 我们的QQ群号
-
评论