文章目录(Table of Contents)
简介
这一篇文章会详细的介绍一下Pytorch中模型构建的方法(构建Block的方法), 注意包含下面的几种形式:
- 自定义一个Block, 直接继承nn.Module;
- 使用Sequential Block的方式进行定义;
- 自定义forward函数;
- 将上面的几种方法进行混合;
除了介绍模型构建的方法, 我们还会介绍对模型中的参数进行不同的操作.
- 参数的获取与操作;
- 参数的初始化操作;
- 参数绑定;
Pytorch模型构建方法
Custom Block
首先是最常用的一种方式, 我们可以通过直接继承nn.Module, 对其进行修改, 来完成我们需要的网络结构. 例如下面是一个全连接网络的实现, 我们只需要完成init的部分, 和forward的部分. 关于参数初始化, 反向传播会由Pytorch框架来完成.
- class MLP(nn.Module):
- # Declare a layer with model parameters. Here, we declare two fully
- # connected layers
- def __init__(self):
- # Call the constructor of the MLP parent class Block to perform the
- # necessary initialization. In this way, other function parameters can
- # also be specified when constructing an instance, such as the model
- # parameter, params, described in the following sections
- super().__init__()
- self.hidden = nn.Linear(20,256) # Hidden layer
- self.out = nn.Linear(256,10) # Output layer
- # Define the forward computation of the model, that is, how to return the
- # required model output based on the input `x`
- def forward(self, x):
- # Note here we use the funtional version of ReLU defined in the
- # nn.functional module.
- return self.out(F.relu(self.hidden(x)))
在自定义init的时候, 我们需要需要首先使用super().init()来调用父类的init方法.
Sequential Block
下面是构建序列结构的方式. 在Pytorch中有默认的构建序列的方式, 我们可以使用nn.Sequential, 如下所示:
- net = nn.Sequential(nn.Linear(20, 256), nn.ReLU(), nn.Linear(256, 10))
同样, 我们也是可以进行自定义. 需要注意的是我们没有直接使用一个list来进行存储, 而是使用Module中的_modules的有序字典类型来进行存储. 这样使用的一个好处是, 在模型参数初始化的时候, 会对_modules进行sub-block同样进行初始化.
- class MySequential(nn.Module):
- def __init__(self, *args):
- super().__init__()
- for block in args:
- # Here, block is an instance of a Module subclass. We save it in
- # the member variable _modules of the Module class, and its type
- # is OrderedDict
- self._modules[block] = block
- def forward(self, x):
- # OrderedDict guarantees that members will be traversed in the order
- # they were added
- for block in self._modules.values():
- x = block(x)
- return x
使用的方法还是和上面的nn.Sequential是一样的, 传入我们需要的Block即可.
- net = MySequential(nn.Linear(20, 256), nn.ReLU(), nn.Linear(256, 10))
Executing Code in the forward Method
上面我们都是直接使用Pytorch中现成的模块. 我们也可以在其中自定义一些操作. 例如可以有Python中的控制流 (control flow); 同时, 我们也可以进行自定义的运算. 例如下面的例子.
- 首先是rand_weight是不需要计算梯度的, 也就是requires_grad=False.
- 同时, 在forward部分, 会有一个while循环.
- class FixedHiddenMLP(nn.Module):
- def __init__(self):
- super().__init__()
- # Random weight parameters that will not compute gradients and
- # therefore keep constant during training
- self.rand_weight = torch.rand((20, 20), requires_grad=False)
- self.linear = nn.Linear(20, 20)
- def forward(self, x):
- x = self.linear(x)
- # Use the constant parameters created, as well as the relu and dot
- # functions
- x = F.relu(torch.mm(x, self.rand_weight) + 1)
- # Reuse the fully connected layer. This is equivalent to sharing
- # parameters with two fully connected layers
- x = self.linear(x)
- # Here in Control flow, we need to call asscalar to return the scalar
- # for comparison
- while x.norm().item() > 1:
- x /= 2
- return x.sum()
Mixed Block
最后我们看一个比较综合的应用, 将上面的几种定义Block的方法都集中起来.
- 总的是通过nn.Sequential来进行连接的.
- 第一个部分, NestMLP也是包含两个部分.
- 接着是一个nn.Linear
- 最后是一个上面定义的, 自定义了forward函数的Block.
- class NestMLP(nn.Module):
- def __init__(self):
- super().__init__()
- self.net = nn.Sequential(nn.Linear(20, 64), nn.ReLU(),
- nn.Linear(64, 32), nn.ReLU())
- self.linear = nn.Linear(32, 16)
- def forward(self, x):
- return self.linear(self.net(x))
- chimera = nn.Sequential(NestMLP(), nn.Linear(16, 20), FixedHiddenMLP())
Pytorch中的自定义Layer
上面我们讲了在Pytorch中, 如何构建整个模型, 这里再着重介绍一下每一个Layer如何进行自定义.
Layer中不包含系数
首先, 我们可以定义一个不包含参数的Layer, 如下面的, 我们是数据的均值为0.
- class CenteredLayer(nn.Module):
- def __init__(self):
- super().__init__()
- def forward(self, x):
- return x - x.mean()
例如, 当我们的输入是(1,2,3,4,5), 此时均值是3的时候, 输出为(-2,-1,0,1,2). 如下面例子所示.
- layer = CenteredLayer()
- layer(torch.FloatTensor([1, 2, 3, 4, 5]))
- """
- tensor([-2., -1., 0., 1., 2.])
- """
我们可以将我们定义的这一层很容易的加到整个网络中去.
- net = nn.Sequential(nn.Linear(8, 128), CenteredLayer())
Layer中包含参数
上面我们看了简单的Layer是如何进行定义的. 现在我们看一下当一个Layer中包含参数, 在训练的时候, 我们需要更新这些参数. 这个时候应该如何进行定义. 下面的例子, 我们实现了一个简单的全连接层的效果.
- class MyLinear(nn.Module):
- def __init__(self, in_units, units):
- super().__init__()
- self.weight = nn.Parameter(torch.randn(in_units, units))
- self.bias = nn.Parameter(torch.randn(units,))
- def forward(self, x):
- return torch.matmul(x, self.weight.data) + self.bias.data
同样, 我们可以使用我们定义的网络层来组成一个模型.
- net = nn.Sequential(MyLinear(64, 8), nn.ReLU(), MyLinear(8, 1))
- net(torch.randn(2, 64))
Pytorch网络参数的相关控制
在这一部分, 我们主要从模型参数的三个主要部分来进行介绍:
- Accessing parameters for debugging, diagnostics, and visualizations. (查看网络的参数)
- Parameter initialization.
- Sharing parameters across different model components.
Parameter Access
简单参数的获取
现在假设我们使用nn.Sequential来构建网络, 如下所示, 我们构建一个简单的全连接网络.
- net = nn.Sequential(nn.Linear(4, 8), nn.ReLU(), nn.Linear(8, 1))
那么, 当我们通过Sequential
来进行定义的时候, 我们可以通过index的方式获得每一层的参数. 下面我们来看一下最后一层的参数. 最后是通过有序字典(OrderedDict)进行返回.
- print(net[2].state_dict())
- """
- OrderedDict([('weight', tensor([[-0.3289, -0.3328, -0.2062, 0.0865, 0.2737, 0.3170, 0.0943, -0.0462]])), ('bias', tensor([0.1746]))])
- """
我们看到第二个是激活函数ReLU, 他是没有系数的, 我们也是同样可以看一下.
- print(net[1].state_dict())
- """
- OrderedDict()
- """
上面只是返回了字典类型, 为了做一些更有用的操作, 我们需要获得他的数字的值(numerical values). 我们通过下面的方式进行获得.
- print(net[2].bias)
- print(net[2].bias.data)
- """
- tensor([0.1746], requires_grad=True)
- tensor([0.1746])
- """
除了获得上面的data之外, 我们还是可以获得gradients, 并对其进行设置. 看下面一个简单的例子.
- net[2].weight.grad == None
所有系数一起操作
有的时候我们需要对所有的系数一起进行操作, 上面的方法就会显得比较麻烦. 那么我们总体看一下整个网络的OrderedDict是什么样子的. 可以看到第一层的key是'0.weight'和'0.bias'. 也就是每一层会在前面加上编号.
- net.state_dict()
- """
- OrderedDict([('0.weight', tensor([[-0.4401, 0.1671, -0.2557, -0.2542],
- [ 0.0476, -0.3741, 0.2795, 0.2561],
- [-0.1753, -0.3761, 0.2565, 0.2287],
- [-0.1435, -0.4030, 0.1671, 0.2304],
- [ 0.1131, 0.4255, -0.1356, 0.4474],
- [ 0.3750, -0.0760, 0.1078, -0.1561],
- [-0.1217, -0.3878, -0.3331, 0.3495],
- [ 0.0281, -0.2158, 0.3175, -0.0070]])),
- ('0.bias', tensor([ 0.2330, 0.2435, -0.3555, -0.3084, 0.0134, -0.4436, -0.4233, 0.0456])),
- ('2.weight', tensor([[-0.3289, -0.3328, -0.2062, 0.0865, 0.2737, 0.3170, 0.0943, -0.0462]])),
- ('2.bias', tensor([0.1746]))])
- """
那么, 此时我们要获取某一层的系数, 就可以先获取整个net的系数, 接着按照key来进行索引. 下面是获得第二层的weight的值, 可以看到和之前获取的是一样的.
- net.state_dict()['2.weight'].data
- """
- tensor([[-0.3289, -0.3328, -0.2062, 0.0865, 0.2737, 0.3170, 0.0943, -0.0462]])
- """
系数初始化(Parameter Initialization)
上面我们介绍了如何获取网络中的系数, 下面我们来看一下如何对其进行初始化的操作. 我们的框架会随机对系数进行初始化, 但是有的时候我们会希望指定方法来进行初始化.
Built-in Initialization
首先我们看一下使用内置的初始话的方法. 也就是修改init的时候系数初始化的方法. 下面我们初始化的方法是:
- 将weight的系数初始化为, 均值=0, 方差=0.01的高斯分布;
- 将bias初始化为0;
- def init_normal(m):
- if type(m) == nn.Linear:
- nn.init.normal_(m.weight, mean=0, std=0.01)
- nn.init.zeros_(m.bias)
- net = nn.Sequential(nn.Linear(4, 8), nn.ReLU(), nn.Linear(8, 1))
- # 对net使用这个初始化的规则
- net.apply(init_normal)
- # 查看系数
- net[0].weight.data[0], net[0].bias.data[0]
- """
- (tensor([ 0.0007, -0.0123, -0.0065, 0.0029]), tensor(0.))
- """
除了上面的初始化的方法之外, 我们还可以将所有的系数都初始化为常数, 下面我们将所有的weight都设置为1, 所有的bias都设置为0:
- def init_constant(m):
- if type(m) == nn.Linear:
- nn.init.constant_(m.weight, 1)
- nn.init.zeros_(m.bias)
- net = nn.Sequential(nn.Linear(4, 8), nn.ReLU(), nn.Linear(8, 1))
- net.apply(init_constant)
- net[0].weight.data[0], net[0].bias.data[0]
- """
- (tensor([1., 1., 1., 1.]), tensor(0.))
- """
除此之外, 我们还可以对不同的layer进行不同的初始化操作, 如下所示:
- def xavier(m):
- if type(m) == nn.Linear:
- torch.nn.init.xavier_uniform_(m.weight)
- def init_42(m):
- if type(m) == nn.Linear:
- torch.nn.init.constant_(m.weight, 42)
- net[0].apply(xavier) # 使用Xavier initializer
- net[2].apply(init_42) # 初始化为常数
- print(net[0].weight.data[0])
- """
- tensor([ 0.0454, -0.1850, 0.4538, 0.4998])
- """
- print(net[2].weight.data)
- """
- tensor([[42., 42., 42., 42., 42., 42., 42., 42.]])
- """
Custom Initialization
有的时候, 有一些初始化的方法是框架没有提供的, 这个时候我们就需要进行自定义操作了. 上面用的初始化的方法, 比如初始化为全0, 全1或是高斯分布, 都是Pytorch中框架自带的. 我们只是对不同的层使用不同的初始化的方法.
但是现在我们要自己定义一个初始化的分布. 例如我们定义一个一下的分布, 用来初始化系数.
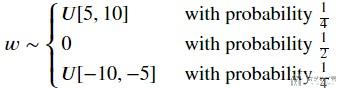
上面可以使用torch.nn.init来进行初始化, 这里我们就需要自行对系数进行操作. 例如下面, 当我们对weight进行初始化之后, 我们需要将[-5,5]之间都设置为0.
- def my_init(m):
- if type(m) == nn.Linear:
- nn.init.uniform_(m.weight, -10, 10)
- # print(m.weight)
- m.weight.data *= m.weight.data.abs() >= 5 # 只保留大于5或者小于-5的系数
- # print(m.weight)
- # 在[-10,10]之间的系数
- """
- ensor([[-1.5157, 5.7350, 3.4442, -3.4822],
- [-4.8276, -4.6641, 3.5532, -9.3350],
- [ 8.3723, 3.6050, 7.3844, -9.5979],
- [-0.7135, 9.4364, 0.9190, 9.9970],
- [-8.1832, -8.9159, 8.3061, 8.8829],
- [ 4.4121, 2.8894, 6.2797, -7.7932],
- [-8.5017, -6.3802, 3.9993, -1.8441],
- [-6.8312, 9.8856, 9.0257, -5.2401]], requires_grad=True)
- """
- # 只取得>5或是<-5
- """
- tensor([[-0.0000, 5.7350, 0.0000, -0.0000],
- [-0.0000, -0.0000, 0.0000, -9.3350],
- [ 8.3723, 0.0000, 7.3844, -9.5979],
- [-0.0000, 9.4364, 0.0000, 9.9970],
- [-8.1832, -8.9159, 8.3061, 8.8829],
- [ 0.0000, 0.0000, 6.2797, -7.7932],
- [-8.5017, -6.3802, 0.0000, -0.0000],
- [-6.8312, 9.8856, 9.0257, -5.2401]], requires_grad=True)
- """
同样, 我们还是使用apply来对目标网络的参数进行初始化.
- net.apply(my_init)
同时, 我们也是可以直接对系数进行修改的.
- net[0].weight.data[:] += 1
- net[0].weight.data[0, 0] = 42
- net[0].weight.data[0]
参数绑定
有的时候, 我们希望不同layer之间是可以共享系数的, 一个简单的方法就是首先在外面定义一层, 接着里面使用同一个block. 下面看一个简单的例子.
- shared = nn.Linear(8, 8)
- net = nn.Sequential(nn.Linear(4, 8), nn.ReLU(),
- shared, nn.ReLU(),
- shared, nn.ReLU(),
- nn.Linear(8, 1))
我们首先定义一个shared层, 接着在net的时候, 其中net[2]和net[4]都是使用的shared. 我们修改其中的一个系数.
- net[2].weight.data[0, 0] = 100
另外一个layer的系数也是会一起进行改变的.
- print(net[2].weight.data[0,0], net[4].weight.data[0,0])
- """
- tensor(100.) tensor(100.)
- """
那么此时这两层的系数是一样的, 我们如何进行反向传播呢. 在实际计算梯度的时候, 会对这两层的梯度值进行累加, 接着一起进行反向传播. (the gradients of the second hidden layer and the third hidden layer are added together during backpropagation.)
- 微信公众号
- 关注微信公众号
-
- QQ群
- 我们的QQ群号
-
评论