文章目录(Table of Contents)
简介
这一篇我们会讲解二分类问题和多分类问题。在「二分类问题」中,我们可以使用 Sigmoid
作为输出, 看成是一类的概率,另一类的概率就是 1-Sogmoid
的输出结果。但是在多分类问题中,我们需要每一类的概率,这个就需要使用 Softmax
。
参考资料
- 关于交叉熵的原理介绍: 熵, 交叉熵, 和KL散度
- 关于 Pytorch 中 CrossEntropyLoss 的介绍: PyTorch中交叉熵的计算-CrossEntropyLoss介绍
- 关于下面
Softmax
的图片来源: Deep Learning — Logistic Classification — using Softmax function - 一个特别好的文章, Understanding softmax and the negative log-likelihood
关于 Softmax 和多分类问题
Softmax 函数介绍
在多分类问题中,我们需要对每一个类别输出概率,同时要保证这些概率和是 1
。这个时候就需要使用 Softmax
函数了。
假设现在的输出是 vi
(这是一个向量),那么每一个对应 softmax
之后的输出如下:
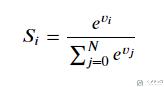
在这里对输入进行指数化,这样可以使得两个输入之间的差距可以扩大。
下面我们看一个 softmax
的例子,并使用 Pytorch
中自带的函数进行测试。
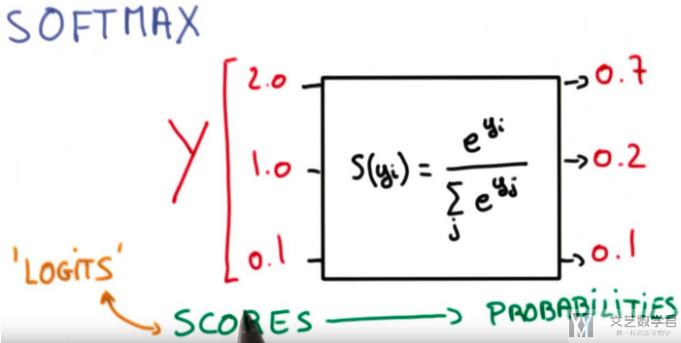
下面看一下使用 Pytorch
的测试结果:
- x = torch.tensor([2, 1, 0.1])
- s = torch.softmax(x, dim=0)
- print('Sotfmax的输出:{}'.format(s))
- print('Sotfmax的输出总和:{}'.format(s.sum().item()))
- """
- Sotfmax的输出:tensor([0.6590, 0.2424, 0.0986])
- Sotfmax的输出总和:1.0000001192092896
- """
对于多组数据来说,其实 softmax
做的就是将一个矩阵的值压缩到 0
到 1
之间。例如下面的例子,测试数据有三类:
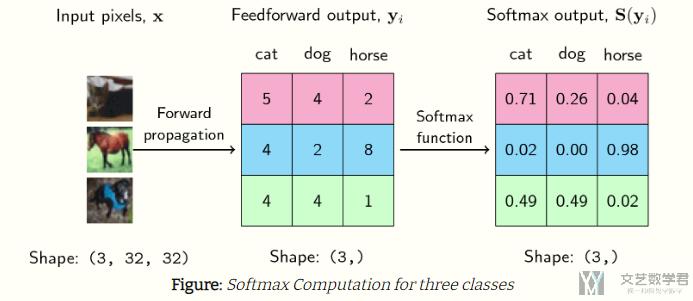
The output of the softmax describes the probability (or if you may, the confidence) of the neural network that a particular sample belongs to a certain class.
Thus, for the first example above, the neural network assigns a confidence of 0.71 that it is a cat, 0.26 that it is a dog, and 0.04 that it is a horse. The same goes for each of the samples above.
交叉熵损失函数
在介绍交叉熵损失函数之前,我们首先看一个例子。现在有一个三分类的问题,一共有三个测试数据,两个测试的模型,他们的结果分别如下所示:
模型一的结果:

模型二的结果:

- 对于两个模型的准确率来说,是一样的,准确率都是
2/3
; - 对于「模型一」来说,他在
数据 1
和数据 2
上,对结果的确定性都不是很确定,都是0.4
,只比0.3
大一点; - 对于「模型二」来说,他的预测结果就表现很肯定,对结果的可能性都是
0.7
,会远远比其他类别要大。
所以可以看到,虽说上面两个模型在准确率上的表现是一样的,但是实际上,模型二会比模型一要更好一些。于是,我们就需要使用一个 loss 函数
,能够反映出上面模型的好坏。这个时候就需要使用交叉熵损失。(关于交叉熵的损失, 可以查看熵, 交叉熵, 和KL散度)
首先关于交叉熵的计算如下所示:

其中:
- p(真实的分布),在实际中也就是 0 和 1,是正确的 label 就是 1,否则就是 0;
- q(预测的概率),模型给出的概率(经过 softmax),都是介于 0-1 之间的数字;
因为 q
是介于 0-1
之间的数字,所以经过 log
之后为负数,然后前面又加了一个负号。如果对正确标签给出的概率越大,那个这个值就会越小(例如 log(0.5)=-0.69
,log(0.99)=-0.01
)。于是整个的 loss
可以定义为下面的样子:
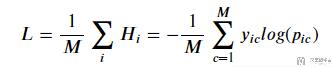
其中:
M
为总的数据的数量;y
是实际标签, 为one-hot
编码;p
为模型输出的概率;
在实际中,因为 y 只有 0 和 1 两种不同的取值,所以 negative log-likelihood 可以化简为下面的式子:
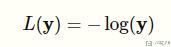
我们画出上面的 L
关于不同输入的图像,可以看到当概率很小的时候,L
会很大;当概率大的时候,L
会很小。也就是说,当网络给正确的结果一个很低的置信度(confidence
)的时候, 此时 L
很大(我们希望 L
越小越好):
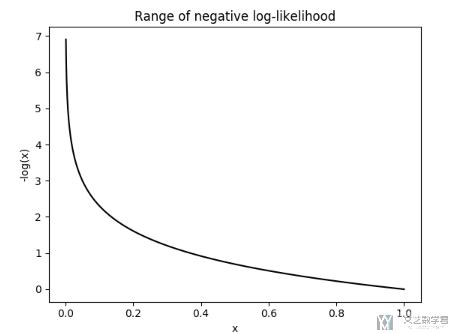
还是上面的例子,在计算完 softmax
之后,我们计算 NLLLoss
。下图中红色的表示正确的是哪一类:
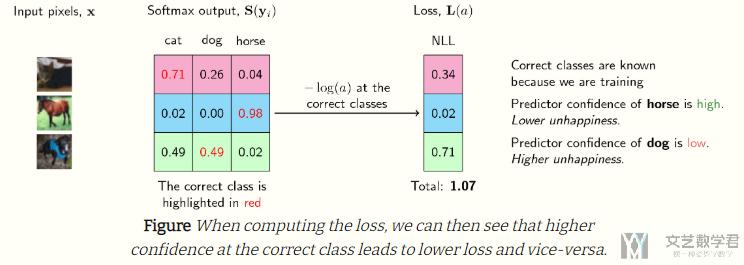
于是,我们使用上面的交叉熵来衡量上面的模型一和模型二。需要注意的是, 在 Pytorch
中,有一个函数是 NLLLoss (negative log likelihood loss
),但是他并没有计算 log
,这个名字起的很奇怪。关于 NLLLoss
的介绍可以查看连接, PyTorch中交叉熵的计算-CrossEntropyLoss介绍
所以,在 Pytorch
,多分类的 loss
我们会使用 nn.CrossEntropyLoss()
,这里面包含了 Logsoftmax+NLLLoss
,他把 Log
的计算和 sotfmax
和在了一起。
我们在这里就自己手动计算 log
,再使用 NLLLoss
来测试一下上面两个模型的好坏。(我们认为是模型二比模型一要好),注意下面的代码,我们对概率值手动计算了 log:
- loss = nn.NLLLoss()
- Y = torch.tensor([2, 1, 0])
- # 模型1对每条数据的预测,每条数据对应三个概率,表示该条数据属于第 i 类的概率值
- model_one_pred = torch.tensor(
- [[0.3, 0.3, 0.4], # predict class 2
- [0.3, 0.4, 0.3], # predict class 1
- [0.1, 0.2, 0.7]]) # predict class 2
- # 模型2对每条数据的预测,每条数据对应三个概率,表示该条数据属于第 i 类的概率值
- model_two_pred = torch.tensor(
- [[0.1, 0.2, 0.7], # predict class 2
- [0.1, 0.7, 0.2], # predict class 1
- [0.4, 0.3, 0.3]]) # predict class 2l1 = loss(Y_pred_good, Y)
- l1 = loss(torch.log(model_one_pred), Y)
- l2 = loss(torch.log(model_two_pred), Y)
- l1, l2
- # > (tensor(1.3784), tensor(0.5432))
可以看到模型一的 loss
比模型二的要大,说明模型二比较好。
关于 Sotfmax 和 NLLLoss 的数学推导
数学推导
上面我们直观的了解了一下 Softamx
和 NLLLoss
的相关内容,这里我们来计算一下他们的导数,更进一步说明这样做的好处。(这一部分的数学推导来自,Understanding softmax and the negative log-likelihood)
首先重写一下关于 softmax
的函数:
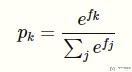
接着是 negative log-likelihood
的函数:
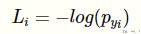
我们是要计算 L
关于 f
的导数,我们可以使用链式法则进行如下的展开:
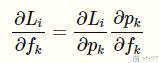
这样就分为了两个部分,我们首先看一下前面一部分:
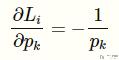
接着我们来计算第二部分。在计算之前,复习一下除法的求导公式:
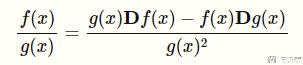
按照上面的方式,对第二部分的式子进行求导:
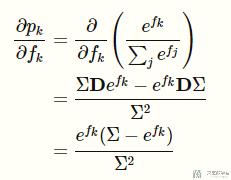
继续对上面的式子进行化简:
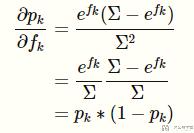
最后我们将第一部分和第二部分结合起来,总的导数为:
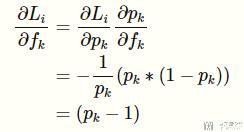
可以看到最终的导数很简单,这也就是为什么通常情况下,我们都是将 softmax
和 negative log likelihood
合起来一起用的,也就是 Pytorch
中的 CrossEntropyLoss
。
下面我们使用 CrossEntropyLoss
来做一下实验,看一下导数是不是和我们推导的是一样的。
使用 CorssEntropyLoss 进行实验
我们还是使用之前的实验数据来进行模拟。下面我们使用了sum,也就是将每一条数据的 loss 进行求和。
- loss = nn.CrossEntropyLoss(reduction='sum')
- # 实际的分类
- Y = torch.tensor([0, 2, 1])
- # 模型一对每条数据的预测,每条数据对应三个概率,表示该条数据属于第 i 类的概率值
- X = torch.tensor(
- [[5, 4, 2], # predict class 2
- [4, 2, 8], # predict class 1
- [4, 4, 1]], dtype=torch.float32, requires_grad=True) # predict class 2
- l = loss(X, Y)
- print('loss:{}'.format(l.item()))
- # >> loss:1.0873291492462158
我们看一下 X 进行 softmax 之后的结果:
- p = nn.Softmax(dim=1)
- print(p(X))
- """
- tensor([[0.7054, 0.2595, 0.0351],
- [0.0179, 0.0024, 0.9796],
- [0.4879, 0.4879, 0.0243]], grad_fn=<SoftmaxBackward>)
- """
可以看到和上面图上是一样的。下面我们再来确认一下上面 loss 的计算。为了自己手动计算 loss。我们需要对 Softmax 的结果求 log。
- -torch.log(p(X))
- """
- tensor([[0.3490, 1.3490, 3.3490],
- [4.0206, 6.0206, 0.0206],
- [0.7177, 0.7177, 3.7177]], grad_fn=<NegBackward>)
- """
于是 loss 的计算也就变成了, loss=0.3490+0.0206+0.7177=1.0873。
上面我们再次确认了 CrossEntropyLoss 是如何进行计算的。下面我们确认上面的求导公式。我们首先进行反向传播,计算X的梯度。
- l.backward() # 反向传播,计算梯度
- print('X的梯度, \n{}'.format(X.grad))
- """
- X的梯度,
- tensor([[-0.2946, 0.2595, 0.0351],
- [ 0.0179, 0.0024, -0.0204],
- [ 0.4879, -0.5121, 0.0243]])
- """
三个负数的值(对应第一条数据的第一个, 第二条数据的第三个, 第三条数据的第二个), 也就是对应label=1的数据, 都是通过(p-1)计算得到的, 其他的都是p (因为y=0, 所以其实在计算NLL Loss的时候没有参与运算).
下面是按照上面的公式推导出来的导数,看到和上面解释的是一样的:
- p(X)-1
- """
- tensor([[-0.2946, -0.7405, -0.9649],
- [-0.9821, -0.9976, -0.0204],
- [-0.5121, -0.5121, -0.9757]], grad_fn=<SubBackward0>)
- """
于是, 使用 CrossEntropyLoss
的总的一个流程如下图所示:
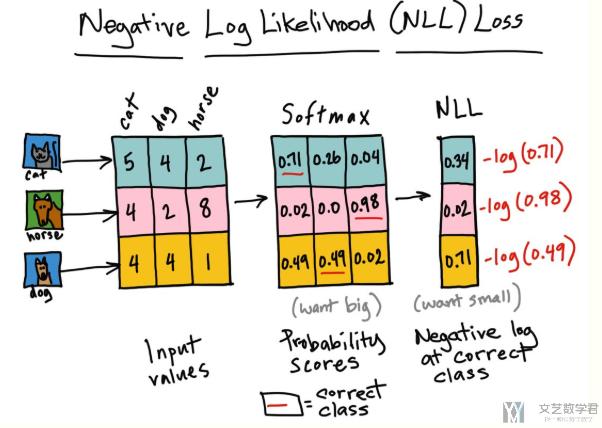
- 微信公众号
- 关注微信公众号
-
- QQ群
- 我们的QQ群号
-
评论